- HOME
- Wow! Cool Laboratory [Researcher Introduction]
- Miki Haseyama
Wow! Cool Laboratory [Researcher Introduction]
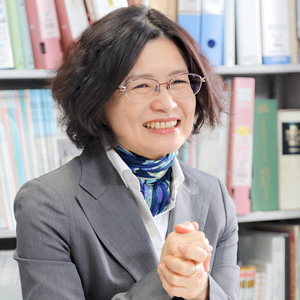
Division of Media and Network Technologies,
Research Group of Information Media Science and Technology,
Laboratory of Media Dynamics
Field of research: image/video/music processing, AI, IoT, big data analysis
Research theme: practical application of multimedia analysis technology based on AI, IoT and big data/complex network analysis
E-mail: miki[a]ist.hokudai.ac.jp
Proposal of AI technologies realizing real world applications based on a high trust relationship with companies
Development of AI technologies with the ability of imagination that can understand and interpret images and videos like humans.
The Laboratory of Media Dynamics led by Professor Miki Haseyama aims at the realization of a next-generation multimedia system that understands images and videos like humans do based on image recognition, image retrieval, video semantic understanding and other technologies. “Understanding like humans do” means a system that reproduces the cognitive function of humans and fundamentally differs from the mechanism of the existing AI. Based on brain science and psychology, the research is carried out to clarify how humans understand and recognize what they see and hear and develop technologies that allow AI to reproduce what humans view.
“For example, the existing AI can find images of cats from the Internet even if it is not trained by humans about cats, because it learns the characteristics of cats from previously accumulated information. Now, if a new species of cat that no one has ever seen appears, can AI recognize it as a cat? Or can AI create the concept of a new species of cat that no one has ever seen? We aim at the development of AI with such “imagination” and technologies creating “awareness” from an enormous amount of data.”
Professor Haseyama says that adding imagination to AI requires embedding a unique layer in the neural network. This is a new attempt to produce imagination and awareness in AI by embedding heterogeneous elements into the conventional cognitive architectures. Specifically, algorithms for learning the relationship between different types of data are added to the architectures of the neural network, and the members of the laboratory freely choose algorithms they are interested in. Of course, this effort does not always produce the results they want, but the process of trial and error accumulates data, providing a clue to the next trial.
“One of the advantages in universities is that there are numerous unique students who can freely think outside of the constraints of society and companies. Adopting elements that individual students are interested in generates diverse ideas, unanticipatedly broadening our perspective. In addition, it is possible to deepen theories and technologies in advanced research environments. This laboratory makes it possible to surely advance projects while maintaining such diversity, attracting attention from many companies.”
Industry-university joint research that makes it possible to continue the cycle of proposition – implementation – verification – sophistication – suggestion
Based on the developed AI technologies, this laboratory is engaged in joint research with various companies and research institutes. The laboratory has developed a system to automatically detect H. pylori infection (note 1) in the medical field, and a system to assist evaluation of the deteriorations of road structures and transmission towers (note 2) in the field of infrastructures. In both cases, AI realizing the learning from accumulated training data was built to assist the detection of infection or determination of repair plans.
Although the accuracy of the systems has not reached 100%, it is notable that these systems have a mechanism that details the reasons for their judgements, and researchers can examine the results. The existing AI only shows the results but does not explain the reason. However, these systems show the reason of the AI’s decisions. Researchers can check parts that AI cannot recognize, or other methods can be used for their decisions. Accordingly, even if the systems are not perfectly accurate, it has been evaluated that they can be applied to practical uses.
“For spreading AI technologies in our society, it is important to provide systems customized to various industries, operations and services. Even if the system is not perfectly accurate, the required accuracies are different for target fields. We can propose technologies suitable for user needs and consider with companies how to set their specifications and required accuracies. While following the PDCA cycles in the real fields, we improve and advance our technologies in the university to develop practical systems. I believe this is ideal industry-academia collaboration.”
In recent years, the laboratory has also carried out studies of eye gaze analysis (note 3) in inspection works for river spaces. By using eye tracking sensors, we monitor several data such as eye movements, areas of focus and eye movement angles to analyze differences between young and skilled engineers.
“If it becomes feasible to represent the know-how of inspections by skilled engineers as image data, even young engineers with little experience can use these data to determine the need of repairs and the degrees of urgency.”
In this laboratory, all research members, including undergraduate students, are required to thoroughly maintain confidentiality and manage information. This creates the trust relationship with companies, helping to secure sufficient data in quality and quantity from the partner companies and the research institutes and to develop systems matched to the needs of target fields.